The world is abuzz about data-driven product management. The truth is that you can’t rely on data to manage your products. Sometimes I feel like a heretic for saying this in the world of modern product management best practices. However, I am only stating a simple and practical fact.
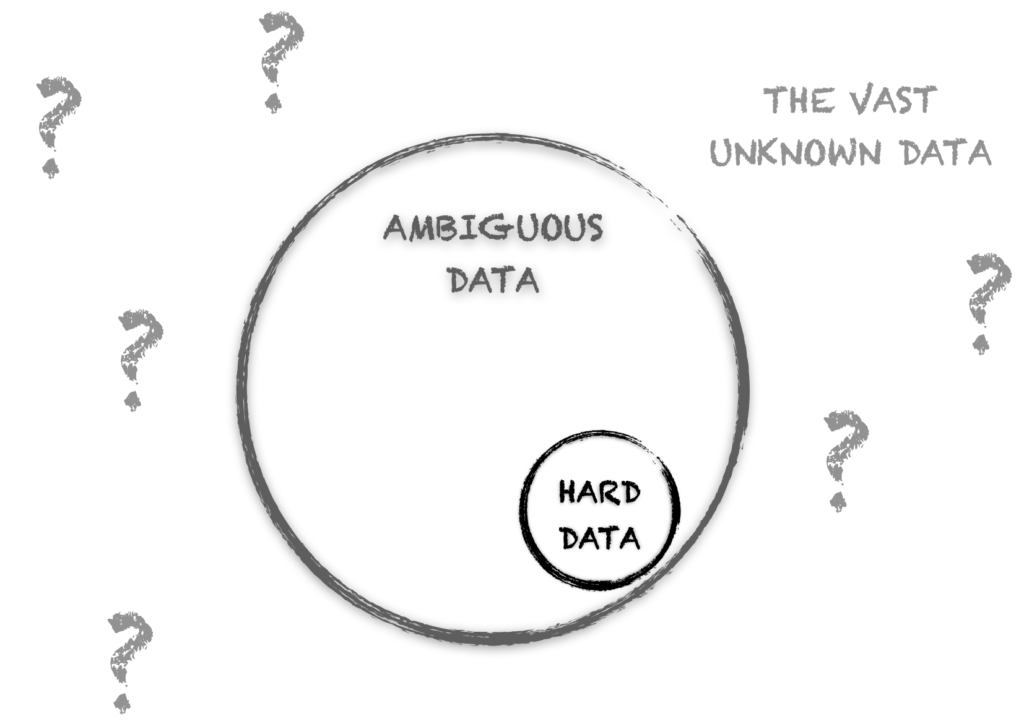
At a high level, data comes in both quantitative and qualitative forms. Quantitative data is most often easiest to gather on existing products and, most especially, SaaS and mobile products. You can also gather quantitative data on markets, competitors, and customers through various forms of research. However, this data is generally imperfect compared to something like behavioral data on your mobile app.
Typically, product managers are looking to find leading indicator data to drive decision making. The acquisition rate in Dave McClure’s AARRR (Pirate Metrics), is one such indicator. A popular example of leveraging quantitative data to improve the acquisition rate would be to perform A/B testing on your application sign-up form to optimize conversions. This is a fantastic use of data but this type of work is just about tuning an existing product capability. It is true you can potentially get a significant lift by tuning your entire application in this manner and should, but it is limited.
Such product tuning activities, I’d argue, should be managed more like DevOps, as an operating practice to improve how you run the existing business.
Qualitative data product teams capture includes win-loss reports, NPS survey feedback, competitor intelligence, and customer support logs. This type of data is not as clear and consequently less actionable. It requires interpretation, analysis, and synthesis with other data sources to both understand root issues and their relative importance.
If you are managing any type of product, at any point in its lifecycle, you encounter a lot of qualitative data. Making sense of this data and converting it into something that is actionable is never straightforward.
Let’s consider an example where your web-based enterprise product is maturing nicely and you are beginning to exploit the product-market fit you achieved last year. Seemingly out of nowhere, a new competitor enters the market that is beating you 4-to-1 in head-to-head new business opportunities. You cannot get access to the competitor’s product. They do not publish their pricing or feature list. A key analyst just placed them next to you in the “leadership” category. You know your existing customers love your product and continue to expand their relationship but new customer growth is now in serious jeopardy.
What do you do? What data do you turn to in order to get answers?
There is no data that will tell you what to do. Frequently, there is not even clear data to tell you why you are losing competitive deals at such a rate. It could be due to product features. Perhaps it has to do with their marketing, pricing, and packaging approach. Maybe they are selling their product as a loss leader to drive revenue in a different part of their business or to simply claim a beachhead against you.
If this sounds familiar to you and you have been banging your head trying to figure out how this data-driven product management stuff applies. You are not alone. These are the real fuzzy challenges that product management teams grapple with at all sorts of companies. It is not a sign of dysfunction or ineptitude at your organization that you don’t have the right data to solve this puzzle.
These uncertainty challenges are pervasive in managing and “Run the Business” activities. If they were not, a computer program could tell you how to run the business. Further, what happens when you are involved with “Change the Business” initiatives? Launching a brand new product. Entering a new market. You are even more reliant on limited and ambiguous data. This absolutely does not mean you should shy away from this work. It is often where the greatest business opportunity lies.
Startups building their very first product are 100% in this area of limited data. Before the first usable release of your product, you have very limited hard data to use for tuning exercises. Once you get the first functional and value-producing release into the customer’s hands, you can use some tuning and optimization techniques to grow product love metrics. In fact, taken to an extreme this feeds into a Product-Led Growth approach to product management. However, before you have that first release you have no hard data. Limited feedback. No referrals. No product to lead the growth.
Now consider new hardware products, on-premises software, complex portfolios of tightly overlapping software solutions involving integrated partner products, or perhaps an ecosystem of IIoT hardware and software services combined to solve highly complex problems. For those in the trenches, know, there is no great source of data available to drive all of your decision-making that others have and you are missing.
This is where data-driven product management hits its limits.
How to move forward in the face of ambiguous data?
Lean UX (Jeff Gothelf) and Lean Enterprise (Jez Humble, Joanne Molesky, Barry O’Reilly) provide some excellent guidance on how to move forward in the face of ambiguous data. At the risk of over-simplifying, they define an approach to move forward through minimal investments in order to gain the feedback (aka data) necessary to increase confidence in the current direction before investing more. You do this by defining hypotheses and developing tests (aka MVPs, bets). You keep doing this until you remove risky assumptions and move into the phase of exploiting your product.
This works great for new products and other investment initiatives. However, you still have to start by making some decisions on what markets to go after. Which customers in those markets to focus on and learn their problems. Where does this starting point come from?
Further, most companies have multiple investment initiatives in mind for new products, extensions, and enhancing existing products and services. How to decide where to allocate time and resources across these alternative ideas in the face of ambiguous data? Even following Lean practices, you can’t do them all.
Your product strategy should be the tool to help articulate the guardrails to guide product team decision-making and investment mix across your portfolio. It should also support a culture of operating in the face of ambiguous data and set a plan that is based on product objectives tied to problem-solving priorities and assumptions.
The product strategy itself must be set into a context of business targets, which are ideally negotiated and not dictated. Yet, you will still find limited hard data at the start to make initial decisions on which initiatives to go after, how much to invest in running the business versus changing the business activities.
From the product managers in the weeds that are responsible for a part of a single product to the Chief Product Officer working on a portfolio product strategy, there will always be decisions made to get the ball rolling based on assumptions. There is where Product Intuition comes in.
Product Intuition is a skill developed by product people through continuous exploration and discovery of the context for which your product decisions are being made. Through continuously exploring the markets, competitors, customers, technology, products, and business factors inherent to your product context, you can build this skill. This is the keystone to making the initial assumptions and hypotheses to enable your plan to be defined and set into motion.
Without that first step guided by Product Intuition, you never get to collect data. Without data, there is no data-driven product management. Therefore, it is critical, that product people continually develop their Product Sense as it can be the difference between accelerating down the right path versus heading into a multitude of dead-ends and pivots.